The Commoditization of Multi-Armed Bandit Experiments for Lifecycle Marketing
How do you search for the right message, timing, and channel to communicate with your customers while simultaneously optimizing for CTR, Orders, CAC, or any of your core metrics? The Multi-Armed Bandit (MAB) could be a practical solution. The catchy name comes from a hypothetical scenario where a casino player tries different slot machines, pulling the arm of each one to find the highest payouts, and then focuses on the machines that offer the greatest ROI. In lifecycle marketing, MAB experiments have inherent advantages over traditional A/B tests, like responding to real-time behavioral patterns, which is great for evergreen campaigns. With recent advances in data processing, it’s an opportune time to consider the history of bandits and how they can be implemented for your business.
The Enigmatic Bandit
The Multi-armed bandit problem was conceived during World War II, but due to its complexity, it was never utilized in a meaningful way. Quote mathematician Peter Whittle, in his 1979 response to "Bandit Processes and Dynamic Allocation Indices" by J.C. Gittins,
"[…] the problem is a classic one; it was formulated during the war, and efforts to solve it so sapped the energies and minds of Allied analysts that the suggestion was made that the problem be dropped over Germany, as the ultimate instrument of intellectual sabotage."
In marketing, individual treatments (ads, messages, etc.) are the analogous arms of the slot machines. Each treatment has an unknown impact on customer engagement and satisfaction. By continuously testing different marketing strategies and reallocating resources to the most effective ones, we can enhance overall engagement and revenue. The foundational paradox of how to make decisions given limited resources and uncertainty applies to many scenarios that we encounter in lifecycle marketing, such as:
— Which notifications should be sent to a user in their first 30 days?
— Which messaging channel should we use to contact the user?
— Which is the best offer to promote to the user?
"Bandit problems embody in essential form a conflict evident in all human action: information versus immediate payoff."
The Contextual Bandit
Decades of research and refinement have yielded robust solutions to the MAB problem, which leading tech companies are increasingly utilizing. When MAB algorithms go beyond the basics by incorporating contextual information like user engagement, location, and feature usage to optimize ROI, they become contextual bandits.
One of the most popular MAB solutions that can iterate with context on top of a core equation is called the epsilon-greedy algorithm. The algorithm balances exploration (epsilon/ϵ) and exploitation (greedy) by selecting a random action with probability ϵ and the best-known action with probability 1-ϵ. After each action, the algorithm updates the estimated rewards for the selected arm based on the observed reward.
Spotify’s Implementation
Spotify is one of the tech titans that has led the way in researching and employing contextual bandits over the past decade. One of their first successful implementations was in personalizing user homepages. By analyzing user features like listening habits, time of day, and location, Spotify continuously learns the optimal playlists to present upon each app launch, maximizing user engagement.
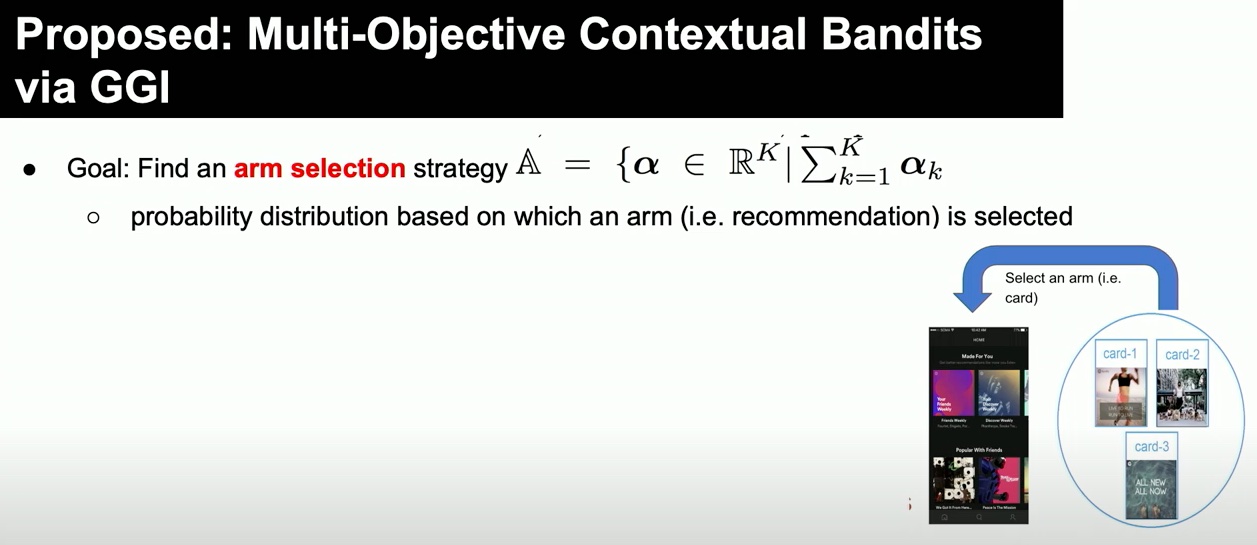
Based on the success of MAB experimentation in the product, Spotify decided to use MABs to take the human guesswork out of determining which push notification to send a user at each stage of their lifecycle. The messages were the arms of the bandit, and the user eligibility of each message was determined by humans — a mix of lifecycle marketers, UX researchers, and engineers. For example, humans, informed by qualitative user research, broadly set guardrails, such as only sending a new release notification if the user is familiar with the artist. A percentage of users in the test were reserved for the “explore” bucket (epsilon/ϵ), where a message (arm) was chosen at random at all times. This bucket was used to continuously train the model, preventing old learnings from going stale and enabling new learnings to gain prominence as they were realized. The remaining percentage of users in the test were in the “exploit” bucket (greedy), which chose the message with the highest predicted ROI for each user. This tactic proved to significantly improve the retention rate for users, even long after the test stopped.

Building this personalization engine at Spotify required significant resources and internal buy-in. Previously, most tech startups didn’t have the resources to replicate this process in-house. However, over the past few years, cross-channel engagement platforms like Braze and Iterable have been incorporating MAB experimentation features, making this powerful technique accessible to resource-constrained companies.
The Democratization of Multi-Armed Bandit Experimentation
Previously, an MAB experiment would take a highly skilled (and costly) team to build, but with cross-channel engagement platforms building out-of-the-box AI features, this superpower is becoming increasingly available for all tech startups and internet technology companies. Many of our platform partners have been implementing pre-packaged MAB solutions into their feature set, such as:
Braze
Braze has been incorporating MAB solutions into their Intelligent Selection feature, which analyzes the performance of an A/B test every 12 hours and automatically adjusts the percentage of users that receive each message variant. This real-time learning approach will rule out underperforming variants and identify high-performing variants faster than a traditional A/B test, ensuring a quicker path to optimal ROI and allowing the model to adapt to new data continuously. Braze client Pizza Hut used Braze’s multi-armed bandit experimentation solution to increase transactions by 30%, revenue by 21%, and profit by 10%.
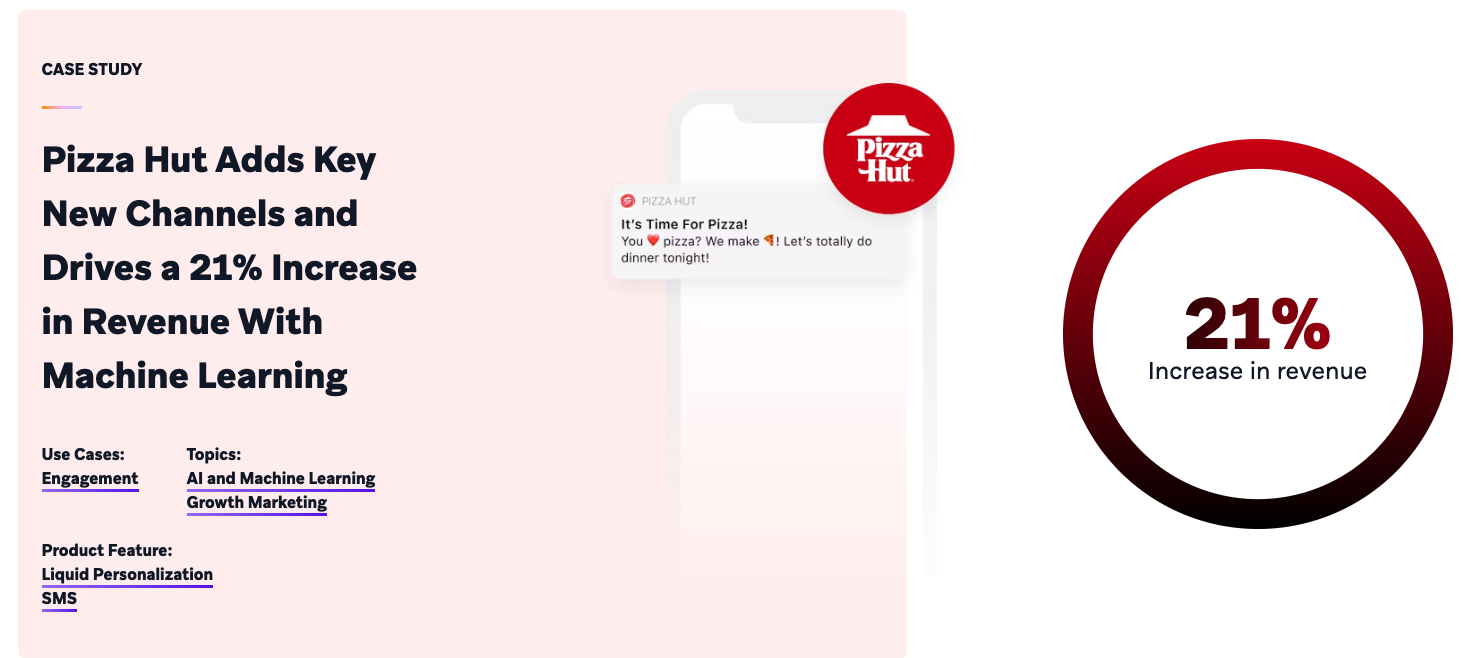
Iterable
Iterable has incorporated multi-armed bandit experimentation into their platform for Triggered Campaigns. They break up the process into two phases. In phase one, Iterable randomly assigns users to one of many variants (including a control holdout) and continues this until it identifies the best-performing variant. In phase two, Iterable sends the currently winning variant to 90% of users, with the other variants sent to the remaining 10% for continued testing. Experiment results are presented in their analytics suite so that operators can glean real-time insights that can be used to develop new, highly effective messages.
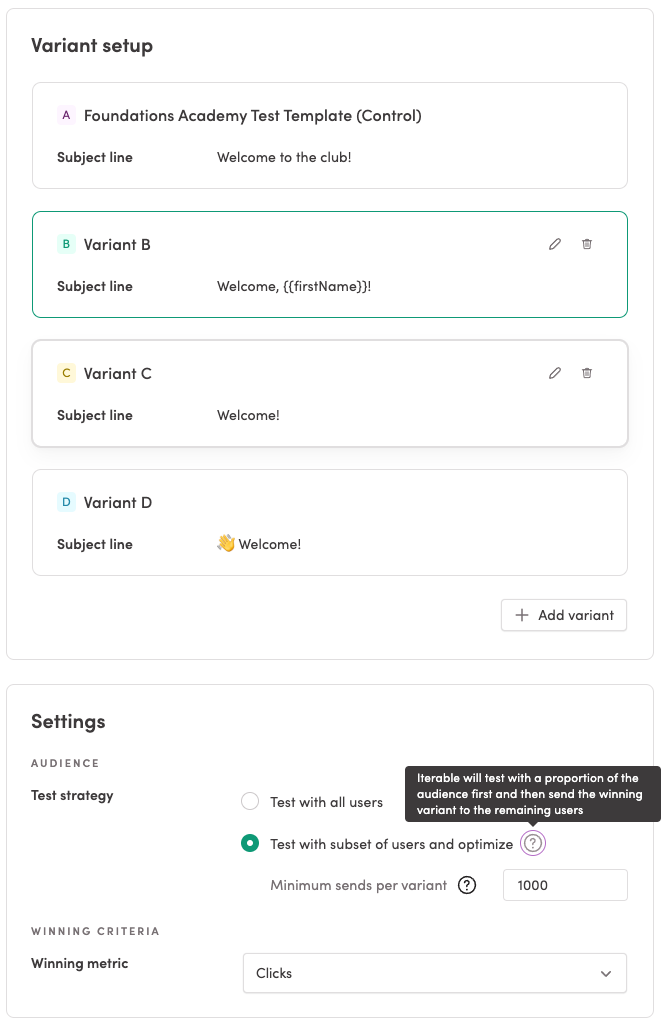
There are several pros and cons to using an out-of-the-box solution created by one of our partners vs. creating a custom solution like Spotify’s. Often, the final decision boils down to resource constraints. Selecting the best platform to run testing for your business could be broken down into its own complex MAB problem, but fortunately, Grohaus has the experience to guide your decision-making and optimize messaging quickly and cost-effectively.
Bandit for Your Business
From 2020-21, Grohaus Founder David Brown was the marketing lead for Spotify's in-house team of experts, which was tasked with using MABs to improve user retention through AI-selected notifications. The project was broken down into three different phases:
Phase 1: Use product and qualitative user insights to create a diverse set of contextualized and personalized messages for users, such as notifications for new album drops by favorite artists. Ensure that there are a number of messages that all users can be eligible for in the scenario where there isn’t a lot of user data (i.e., a very new user or a long-lapsed user). Finally, strive to create repeatable messages that don’t lose appeal after every reuse — personalized messages that can dynamically show a new variable each time: “{artist_name} just released a new album. Tap here to listen.” Repeatable messages can be some of the strongest and make the process of creating messages more efficient.
Phase 2: Implement the MAB experiment by:
A) Determining the KPI you’re optimizing for (i.e., second purchase)
B) Identifying the test audience (i.e., recent first-time purchasers)
C) Determining the sample size of the test audience if you have a big enough database of users
D) Identifying the set of messages that will be in the test
In Spotify’s case, marketers partnered with data scientists and engineers to help determine A, B, and C while still maintaining influence over what messages would be in the test, and what UX guardrails should be considered (D). Braze and Iterable’s out-of-the-box solutions provide easy-to-follow guides on properly implementing an experiment through each of the four steps above.
Phase 3: Monitor the results as winning variants gain statistical significance. Look at the ranking order to glean important insights about what users in your audience are responding better to at a given time. Use those insights to help inform new message production and determine which messages to remove from the test. Be careful about how and when to remove underperforming messages, as seasonality or trending scenarios (like a Drake vs. Kendrick battle) may impact the current results, and allow the ranking to change organically over time.
Previously, only large companies with substantial resources, such as Spotify, could run MAB experiments at scale. Now, with the advent of AI, you can partner with Grohaus to implement game-changing MAB experiments that would have required a whole department just two years ago.
Contact us for a consultation about how Grohaus can help you implement MAB experiments and increase the ROI of your lifecycle marketing program.
Interested in multi-armed bandits for lifecycle marketing?
Get practical advice on how to properly set up MAB experiments that unlock growth.
Book a Free Consultation